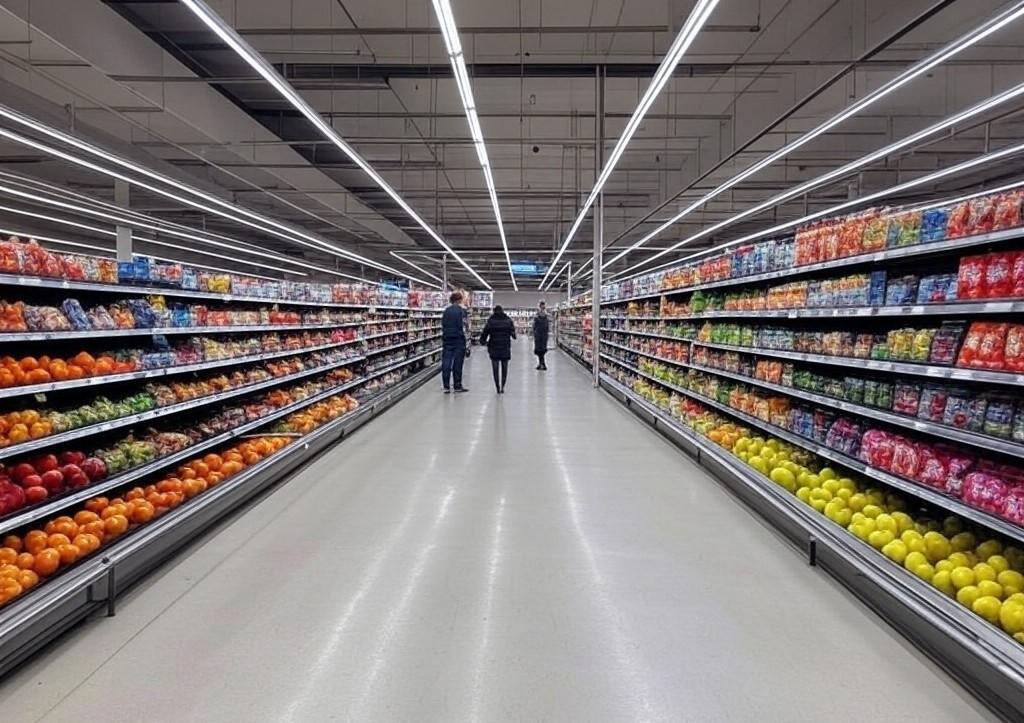
Executive Summary
The Smart Supermarket Project aims to revolutionize traditional grocery shopping by integrating AI-driven automation, IoT-enabled inventory systems, and data analytics to enhance customer experience, optimize operations, and reduce waste. This study evaluates the viability of establishing a tech-driven supermarket that offers personalized shopping, contactless checkout, and sustainable practices. Key findings suggest strong market potential due to rising demand for convenience and tech-integrated retail, though challenges like high upfront costs and consumer tech adoption barriers require strategic mitigation.
Market Feasibility
Industry Trends
Growth Drivers:
The global smart retail market is projected to reach $67 billion by 2028 (CAGR: 24.3%).
Post-pandemic demand for contactless shopping and automated checkout.
Increasing preference for personalized recommendations and eco-friendly practices.
Target Market:
Tech-savvy urban consumers (ages 25–45) seeking convenience.
Busy professionals valuing time-efficient shopping.
Sustainability-focused shoppers prioritizing zero-waste and organic products.
Competitive Landscape
Strengths:
Differentiation via AI-powered personalization and IoT inventory management.
Limited direct competition from traditional supermarkets.
Threats:
Emerging competitors like Amazon Go and Alibaba’s Hema Fresh.
Resistance from older demographics unfamiliar with tech-driven shopping.
Technical Feasibility
Core Technologies
AI/ML Algorithms:
Personalized product recommendations based on purchase history.
Predictive analytics for demand forecasting and waste reduction.
IoT Devices:
Smart shelves with weight sensors and RFID tags for real-time inventory tracking.
Temperature-controlled storage for perishables.
Mobile App:
Features: Digital shopping lists, aisle navigation, and contactless payments.
Automated Checkout:
Computer vision and sensor fusion for “grab-and-go” shopping.
Infrastructure Requirements
Cloud Platform: Centralized data storage for customer profiles and inventory.
In-Store Tech: Installation of smart shelves, cameras, and self-checkout kiosks.
Cybersecurity: Protect customer data and payment systems (GDPR/CCPA compliance).
Challenges
High initial investment in IoT hardware and AI software.
Integration with existing supply chain and vendor systems.
Financial Feasibility
Initial Investment
Cost Category | Estimated Cost |
---|---|
Technology Development | 1.2M |
IoT Hardware & Automation | 800,000 |
Store Setup & Renovation | 500,000 |
Marketing & Launch | 300,000 |
Operational Expenses (Y1) | 600,000 |
Total | 3.4M |
Revenue Streams
Product Sales: Revenue from grocery and household items.
Subscription Services: Premium memberships for express delivery and discounts.
Data Monetization: Aggregated shopping insights for brands (opt-in).
Ad Revenue: In-app promotions and in-store digital ads.
Financial Projections
Metric | Year 1 | Year 2 | Year 3 |
---|---|---|---|
Revenue | 4.5M | 8M | 12M |
Net Profit | -1M | 1.2M | 3.5M |
Break-Even | Achieved by Q2 of Year 3 |
Operational Feasibility
Key Requirements
Location: Urban area with high foot traffic and tech adoption (e.g., city center).
Staffing:
Tech support for IoT/AI systems.
Customer service teams for in-store assistance.
Data analysts for inventory and consumer insights.
Supply Chain: Partnerships with local farmers and eco-friendly suppliers.
Process Workflow
Entry: Customers scan a QR code via the app to enter.
Shopping: Smart shelves update inventory in real-time; app guides customers to items.
Checkout: Automated system detects items in carts and processes payment via app.
Delivery: Optional same-day delivery for app users.
Legal & Regulatory Feasibility
Licensing: Retail operating licenses, food safety certifications.
Data Privacy: Compliance with GDPR, CCPA, and biometric data laws (for facial recognition).
Liability Insurance: Coverage for tech malfunctions or data breaches.
Risk Assessment & Mitigation
Risk | Mitigation Strategy |
---|---|
High Initial Costs | Seek investors or government grants for smart retail initiatives. |
Tech Adoption Resistance | Offer in-store tutorials and discounts for first-time app users. |
Supply Chain Disruptions | Diversify suppliers and use AI for demand forecasting. |
Cybersecurity Threats | Implement end-to-end encryption and regular audits. |
Social Impact
Reduced Waste: AI-driven demand forecasting cuts overstocking by 30%.
Accessibility: Voice-assisted app features for visually impaired shoppers.
Job Creation: New roles in tech maintenance, data analysis, and sustainability management.
SWOT Analysis
Strengths | Weaknesses |
---|---|
Cutting-edge tech integration | High upfront investment |
Personalized shopping experience | Complexity of IoT maintenance |
Opportunities | Threats |
Growing demand for contactless retail | Competition from e-commerce giants |
Partnerships with eco-brands | Regulatory changes impacting data usage |
Recommendations
Pilot Testing: Launch a small-scale store in a tech hub (e.g., San Francisco, Singapore).
Phased Rollout: Prioritize core features (automated checkout, smart shelves) before expanding.
Consumer Education: Host workshops to familiarize shoppers with app and IoT features.
Funding Strategy: Target venture capital firms specializing in retail tech or smart cities.
Conclusion
The Smart Supermarket Project is technically viable and aligns with global trends toward automation, personalization, and sustainability. While the initial investment is significant, the long-term ROI is promising, with projected revenue reaching 12M by Year 3. By addressing risks through strategic partnerships, consumer education, and robust tech infrastructure, the project can redefine modern grocery shopping and set a benchmark for smart retail innovation.
Next Steps:
Finalize pilot location and tech vendor contracts.
Develop MVP (Minimum Viable Product) for beta testing.
Initiate fundraising and partnership discussions.
This feasibility study confirms the Smart Supermarket’s potential to disrupt traditional retail while delivering value to consumers, investors, and the environment.
![]() | ![]() | ![]() |